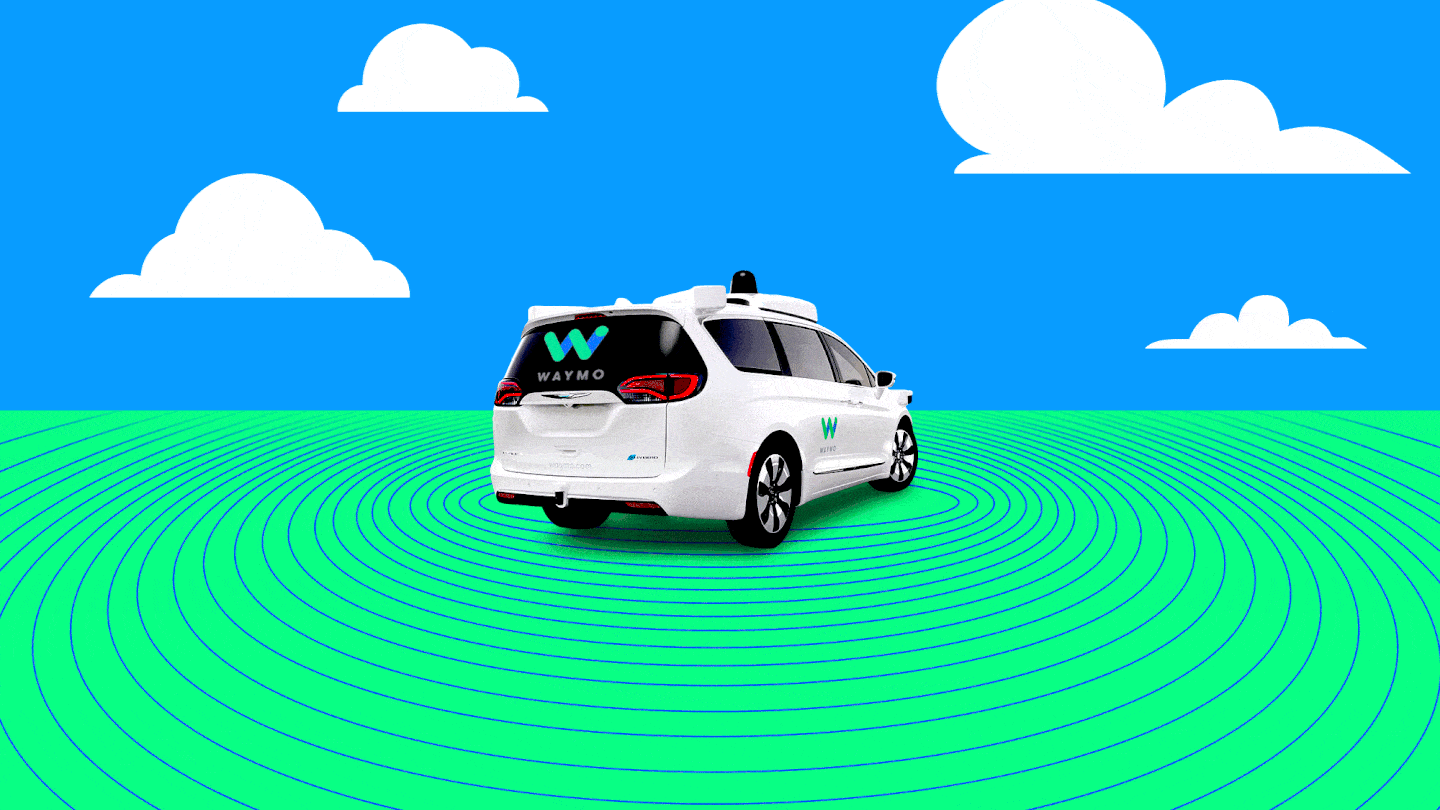
Inside Waymo's Secret World for Training Self-Driving Cars
An exclusive look at how Alphabet understands its most ambitious artificial intelligence project
In a corner of Alphabet’s campus, there is a team working on a piece of software that may be the key to self-driving cars. No journalist has ever seen it in action until now. They call it Carcraft, after the popular game World of Warcraft.
The software’s creator, a shaggy-haired, baby-faced young engineer named James Stout, is sitting next to me in the headphones-on quiet of the open-plan office. On the screen is a virtual representation of a roundabout. To human eyes, it is not much to look at: a simple line drawing rendered onto a road-textured background. We see a self-driving Chrysler Pacifica at medium resolution and a simple wireframe box indicating the presence of another vehicle.
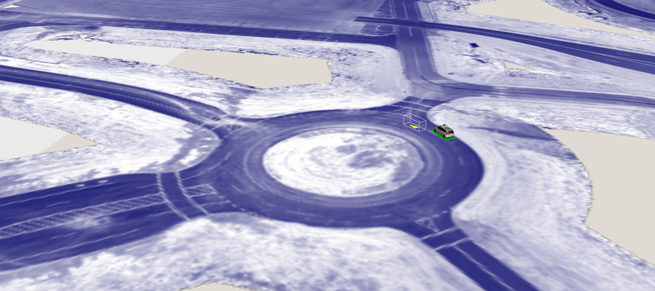
Months ago, a self-driving car team encountered a roundabout like this in Texas. The speed and complexity of the situation flummoxed the car, so they decided to build a look-alike strip of physical pavement at a test facility. And what I’m looking at is the third step in the learning process: the digitization of the real-world driving. Here, a single real-world driving maneuver—like one car cutting off the other on a roundabout—can be amplified into thousands of simulated scenarios that probe the edges of the car’s capabilities.
Scenarios like this form the base for the company’s powerful simulation apparatus. “The vast majority of work done—new feature work—is motivated by stuff seen in simulation,” Stout tells me. This is the tool that’s accelerated the development of autonomous vehicles at Waymo, which Alphabet (née Google) spun out of its “moon-shot” research wing, X, in December of 2016.
If Waymo can deliver fully autonomous vehicles in the next few years, Carcraft should be remembered as a virtual world that had an outsized role in reshaping the actual world on which it is based.
Originally developed as a way to “play back” scenes that the cars experienced while driving on public roads, Carcraft, and simulation generally, have taken on an ever-larger role within the self-driving program.
At any time, there are now 25,000 virtual self-driving cars making their way through fully modeled versions of Austin, Mountain View, and Phoenix, as well as test-track scenarios. Waymo might simulate driving down a particularly tricky road hundreds of thousands of times in a single day. Collectively, they now drive 8 million miles per day in the virtual world. In 2016, they logged 2.5 billion virtual miles versus a little over 3 million miles by Google’s IRL self-driving cars that run on public roads. And crucially, the virtual miles focus on what Waymo people invariably call “interesting” miles in which they might learn something new. These are not boring highway commuter miles.
The simulations are part of an intricate process that Waymo has developed. They’ve tightly interwoven the millions of miles their cars have traveled on public roads with a “structured testing” program they conduct at a secret base in the Central Valley they call Castle.
Waymo has never unveiled this system before. The miles they drive on regular roads show them areas where they need extra practice. They carve the spaces they need into the earth at Castle, which lets them run thousands of different scenarios in situ. And in both kinds of real-world testing, their cars capture enough data to create full digital recreations at any point in the future. In that virtual space, they can unhitch from the limits of real life and create thousands of variations of any single scenario, and then run a digital car through all of them. As the driving software improves, it’s downloaded back into the physical cars, which can drive more and harder miles, and the loop begins again.
To get to Castle, you drive east from San Francisco Bay and south on 99, the Central Valley highway that runs south to Fresno. Cornfields abut subdevelopments; the horizon disappears behind agricultural haze. It’s 30 degrees hotter than San Francisco and so flat that the grade of this “earthen sea,” as John McPhee called it, can only be measured with lasers. You exit near the small town of Atwater, once the home of the Castle Air Force Base, which used to employ 6,000 people to service the B-52 program. Now, it’s on the northern edge of the small Merced metro area, where unemployment broke 20 percent in the early 2010s, and still rarely dips below 10 percent. Forty percent of the people around here speak Spanish. We cross some railroad tracks and swing onto the 1,621 acres of the old base, which now hosts everything from Merced County Animal Control to the U.S. Penitentiary, Atwater.
The directions in my phone are not pointed to an address, but a set of GPS coordinates. We proceed along a tall opaque green fence until Google Maps tells us to stop. There’s nothing to indicate that there’s even a gate. It just looks like another section of fence, but my Waymo host is confident. And sure enough: A security guard appears and slips out a widening crack in the fence to check our credentials.
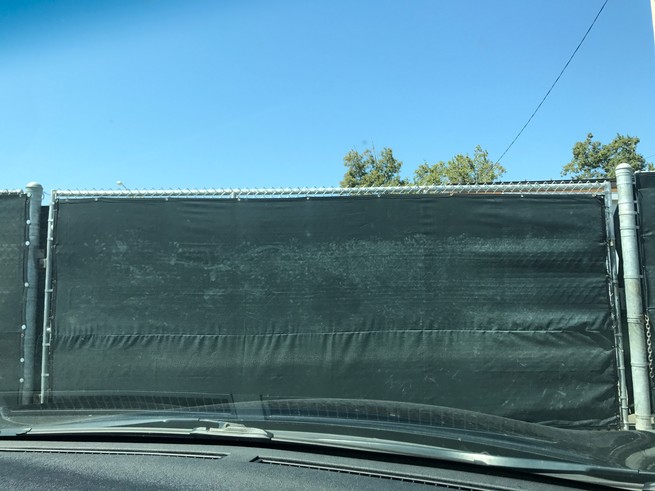
The fence parts and we drive into a bustling little campus. Young people in shorts and hats walk to and fro. There are portable buildings, domed garages, and—in the parking lot of the main building—self-driving cars. This is a place where there are several types of autonomous vehicle: the Lexus models that you’re most likely to see on public roads, the Priuses that they’ve retired, and the new Chrysler Pacifica minivans.
The self-driving cars are easy to pick out. They’re studded with sensors. The most prominent are the laser scanners (usually called LIDARs) on the tops of the cars. But the Pacificas also have smaller beer-can-sized LIDARs spinning near their side mirrors. And they have radars at the back which look disturbingly like white Shrek ears.
When a car’s sensors are engaged, even while parked, the spinning LIDARs make an odd sound. It’s somewhere between a whine and a whomp, unpleasant only because it’s so novel that my ears can’t filter it out like the rest of the car noises that I’ve grown up with.
There is one even more special car parked across the street from the main building. All over it, there are X’s of different sizes applied in red duct tape. That’s the Level Four car. The levels are Society of Automotive Engineers designations for the amount of autonomy that the car has. Most of what we hear about on the roads is Level One or Level Two, meant to allow for smart cruise control on highways. But the red-X car is a whole other animal. Not only is it fully autonomous, but it cannot be driven by the humans inside it, so they don’t want to get it mixed up with their other cars.
As we pull into the parking lot, there are whiffs of Manhattan Project, of scientific outpost, of tech startup. Inside the main building, a classroom-sized portable, I meet the motive force behind this remarkable place. Her name is Steph Villegas.
Villegas wears a long, fitted white collared shirt, artfully torn jeans, and gray knit sneakers, every bit as fashionable as her pre-Google job at the San Francisco boutique Azalea might suggest. She grew up in the East Bay suburbs on the other side of the hills from Berkeley and was a fine-arts major at University of California, Berkeley before finding her way into the self-driving car program in 2011.
“You were a driver?” I ask.
“Always a driver,” Villegas says.
She spent countless hours going up and down 101 and 280, the highways that lead between San Francisco and Mountain View. Like the rest of the drivers, she came to develop a feel for how the cars performed on the open road. And this came to be seen as an important kind of knowledge within the self-driving program. They developed an intuition about what might be hard for the cars. “Doing some testing on newer software and having a bit of tenure on the team, I began to think about ways that we could potentially challenge the system,” she tells me.
So, Villegas and some engineers began to cook up and stage rare scenarios that might allow them to test new behaviors in a controlled way. They started to commandeer the parking lot across from Shoreline Amphitheater, stationing people at all the entrances to make sure only approved Googlers were there.
“That’s where it started,” she says. “It was me and a few drivers every week. We’d come up with a group of things that we wanted to test, get our supplies in a truck, and drive the truck down to the lot and run the tests.”
These became the first structured tests in the self-driving program. It turns out that the hard part is not really the what-if-a-zombie-is-eating-a-person-in-the-road scenarios people dream up, but proceeding confidently and reliably like a human driver within the endless variation of normal traffic.
Villegas started gathering props from wherever she could find them: dummies, cones, fake plants, kids’ toys, skateboards, tricycles, dolls, balls, doodads. All of them went into the prop stash. (Eventually, the props were stored in a tent, and now at Castle, in a whole storage unit.)
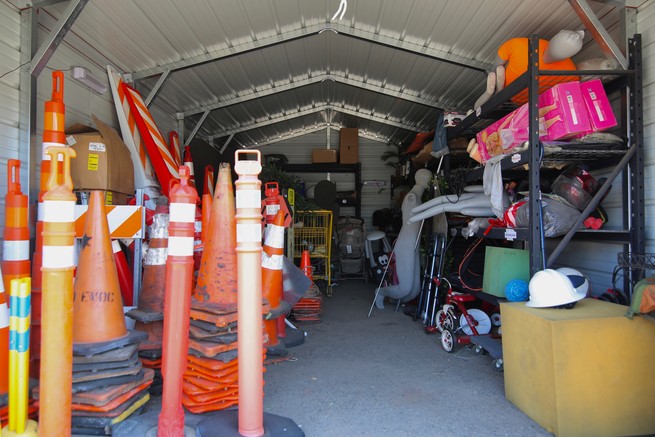
But there were problems. They wanted to drive faster and use streetlights and stop signs. And the concert season at Shoreline Amphitheater regularly threw kinks in their plans. “It was like, ‘Well, Metallica is coming, so we’re gonna have to hit the road,’” she says.
They needed a base, a secret base. And that’s what Castle provided. They signed a lease and started to build out their dream fake city. “We made conscious decisions in designing to make residential streets, expressway-style streets, cul-de-sacs, parking lots, things like that,” she says, “so we’d have a representative concentration of features that we could drive around.”
We walk from the main trailer office to her car. She hands me a map as we pull away to travel the site. “Like at Disneyland, so you can follow along,” she says. The map has been meticulously constructed. In one corner, there is a Vegas-style sign that says, “Welcome to Fabulous Castle, California.” The different sections of the campus even have their own naming conventions. In the piece we’re traveling through, each road is named after a famous car (DeLorean, Bullitt) or after a car (e.g., Barbaro) from the original Prius fleet in the early days of the program.
We pass by a cluster of pinkish buildings, the old military dormitories, one of which has been renovated: That’s where the Waymo people sleep when they can’t make it back to the Bay. Other than that, there are no buildings in the testing area. It is truly a city for robotic cars: All that matters is what’s on and directly abutting the asphalt.
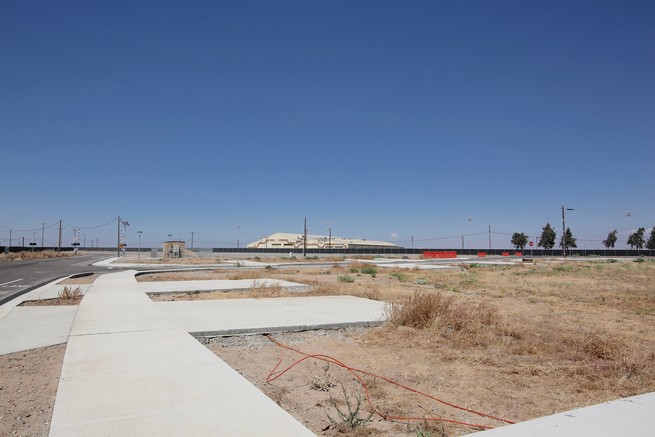
As a human, it feels like a video-game level without the non-player characters. It’s uncanny to pass from boulevards to neighborhood-ish streets with cement driveways to suburban intersections, minus the buildings we associate with these places. I keep catching glimpses of roads I feel like I’ve traveled.
We pull up to a large, two-lane roundabout. In the center, there is a circle of white fencing. “This roundabout was specifically installed after we experienced a multilane roundabout in Austin, Texas,” Villegas says. “We initially had a single-lane roundabout and were like, ‘Oh, we’ve got it. We’ve got it covered.’ And then we encountered a multi-lane and were like, ‘Horse of a different color! Thanks, Texas.’ So, we installed this bad boy.”
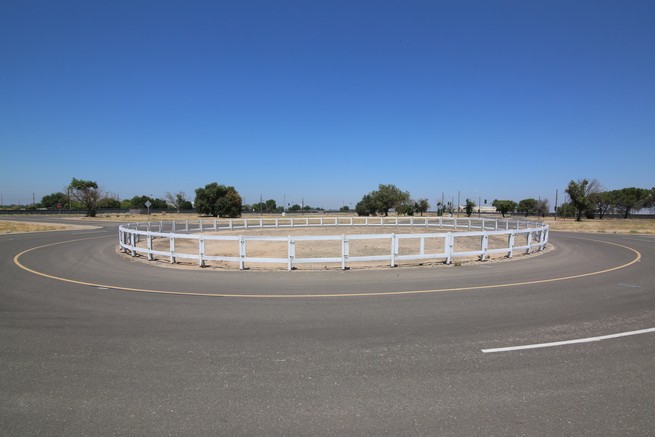
We stop as Villegas gazes at one piece of the new addition: Two car lanes and a bike lane run past parallel parking abutting a grass patch. “I was really keen on installing something with parallel parking along it. Something like this happens in suburban downtowns. Walnut Creek. Mountain View. Palo Alto,” she says. “People are coming out of storefronts or a park. People are walking between cars, maybe crossing the street carrying stuff.” The lane was like a shard of her own memory that she’s embedded in the earth in asphalt and concrete, which will make its way into a more abstract form, an improved ability for a robot to handle her home terrain.
She drives me back to the main office and we hop into a self-driving van, one of the Chrysler Pacificas. Our “left-seat” driver is Brandon Cain. His “right-seat” co-driver in the passenger seat will track the car’s performance on a laptop using software called XView.
And then there are the test assistants, who they call “foxes,” a sobriquet that evolved from the word “faux.” They drive cars, create traffic, act as pedestrians, ride bikes, hold stop signs. They are actors, more or less, whose audience is the car.
The first test we’re gonna do is a “simple pass and cut-in,” but at high speed, which in this context means 45 miles per hour. We set up going straight on a wide road they call Autobahn.
After the fox cuts us off, the Waymo car will brake and the team will check a key data point: our deceleration. They are trying to generate scenarios that cause the car to have to brake hard. How hard? Somewhere between a “rats, not gonna make the light” hard stop and “my armpits started involuntarily sweating and my phone flew onto the floor” really hard stop.
Let me say something ridiculous: This is not my first trip in a self-driving vehicle. In the past, I’ve taken two different autonomous rides: first, in one of the Lexus SUVs, which drove me through the streets of Mountain View, and second, in Google’s cute little Firefly, which bopped around the roof of a Google building. They were both unremarkable rides, which was the point.
But, this is different. These are two fast-moving cars, one of which is supposed to cut us off with a move that will be, to use the Waymo term of art, “spicy.”
It’s time to go. Cain gets us moving and with a little chime, the car says, “Autodriving.” The other car approaches and cuts us off like a Porsche driver trying to beat us to an exit. We brake hard and fast and smooth. I’m impressed.
Then they check the deceleration numbers and realize that we had not braked nearly hard enough. We have to do it again. And again. And again. The other car cuts us off at different angles and with different approaches. They call this getting “coverage.”
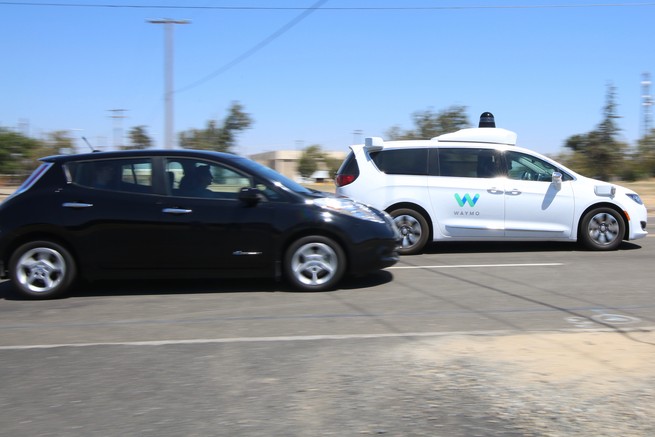
We go through three other tests: high-speed merges, encountering a car that’s backing out of a driveway while a third blocks the autonomous vehicle’s view, and smoothly rolling to a stop when pedestrians toss a basketball into our path. Each is impressive in its own way, but that cut-off test is the one that sticks with me.
As we line up for another run, Cain shifts in his seat. “Have you ever seen Pacific Rim?” Cain asks me. You know the Guillermo del Toro movie where the guys get synced up with huge robot suits to battle monsters. “I’m trying to get in sync with the car. We share some thoughts.”
I ask Cain to explain what he actually means by syncing with the car. “I’m trying to adjust to the weight difference of people in the car,” he says. “Being in the car a lot, I can feel what the car is doing—it sounds weird, but—with my butt. I kinda know what it wants to do.”
Far from the haze and heat of Castle, there is Google’s comfy headquarters in Mountain View. I’ve come to visit Waymo’s engineers, who are technically housed inside X, which you may know as Google X, the long-term, high-risk research wing of the company. In 2015, when Google restructured itself into a conglomerate called Alphabet, X dropped the Google from its name (their website is literally X.company). A year after the big restructuring, X/Alphabet decided to “graduate” the autonomous vehicle program into its own company as it had done with several other projects before, and that company is Waymo. Waymo is like Google’s child, once removed, or something.
So, Waymo’s offices are still inside the mother ship, though, like two cliques slowly sorting themselves out, the Waymo people all sit together now, I’m told.
The X/Waymo building is large and airy. There are prototypes of Project Wing’s flying drones hanging around. I catch a bit of the cute little Firefly car the company built. (“There’s something sweet about something you build yourself,” Villegas had said back at Castle. “But they had no A/C, so I don’t miss them.”)
Up from the cafeteria, tucked in a corner of a wing, is the Waymo simulation cluster. Here, everyone seems to have Carcraft and XView on their screens. Polygons on black backgrounds abound. These are the people creating the virtual worlds that Waymo’s cars drive through.
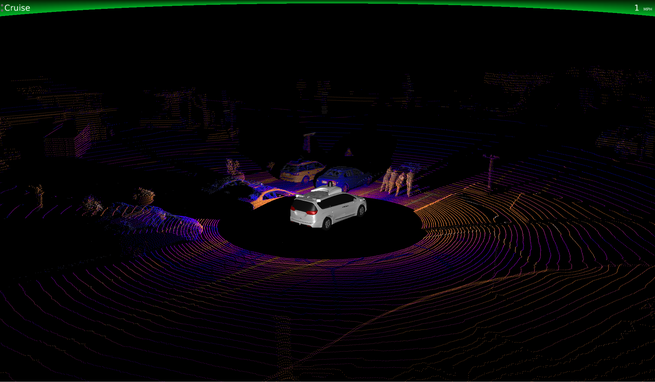
Waiting for me is James Stout, Carcraft’s creator. He’s never gotten to speak publicly about his project and his enthusiasm spills out. Carcraft is his child.
“I was just browsing through job posts and I saw that the self-driving car team was hiring,” he says. “I couldn’t believe that they just had a job posting up.” He got on the team and immediately started building the tool that now powers 8 million virtual miles per day.
Back then, they primarily used the tool to see what their cars would have done in tricky situations in which human drivers have taken over control of the car. And they started making scenarios from these moments. “It quickly became clear that this was a really useful thing and we could build a lot out of this,” Stout says. The spatial extent of Carcraft’s capabilities grew to include whole cities, the number of cars grew into a huge virtual fleet.
Stout brings in Elena Kolarov, the head of what they call their “scenario maintenance” team to run the controls. She’s got two screens in front of her. On the right, she has up XView, the screen that shows what the car is “seeing.” The car uses cameras, radar, and laser scanning to identify objects in its field of view—and it represents them in the software as little wireframe shapes, outlines of the real world.
Green lines run out from the shapes to show the possible ways the car anticipates the objects could move. At the bottom, there is an image strip that displays what the regular (i.e., visible-light) cameras on the car captured. Kolarov can also turn on the data returned by the laser scanner (LIDAR), which is displayed in orange and purple points.
We see a playback of a real merge on the roundabout at Castle. Kolarov switches into a simulated version. It looks the same, but it’s no longer a data log but a new situation the car has to solve. The only difference is that at the top of the XView screen it says “Simulation” in big red letters. Stout says that they had to add that in because people were confusing simulation for reality.
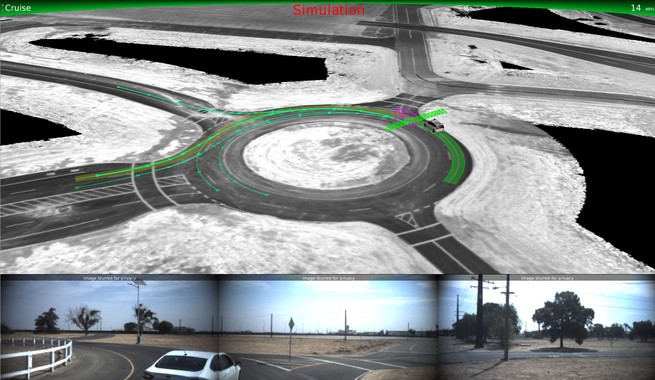
They load up another scenario. This one is in Phoenix. Kolarov zooms out to show the model they have of the city. For the whole place, they’ve got “where all the lanes are, which lanes lead into other lanes, where stop signs are, where traffic lights are, where curbs are, where the center of the lane is, sort of everything you need to know,” Stout says.
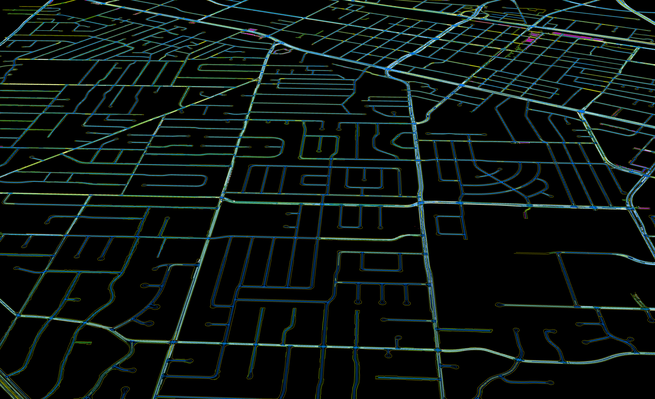
We zoom back in on a single four-way stop somewhere near Phoenix. Then Kolarov starts dropping in synthetic cars and pedestrians and cyclists.
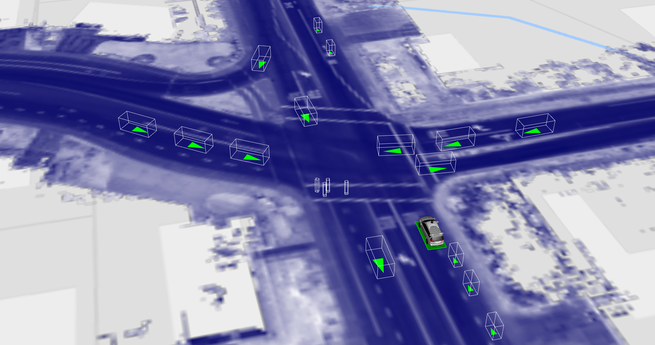
With a hot key press, the objects on the screen begin to move. Cars act like cars, driving in their lanes, turning. Cyclists act like cyclists. Their logic has been modeled from the millions of miles of public-road driving the team has done. Underneath it all, there is that hyper-detailed map of the world and models for the physics of the different agents in the scene. They have modeled both the rubber and the road.
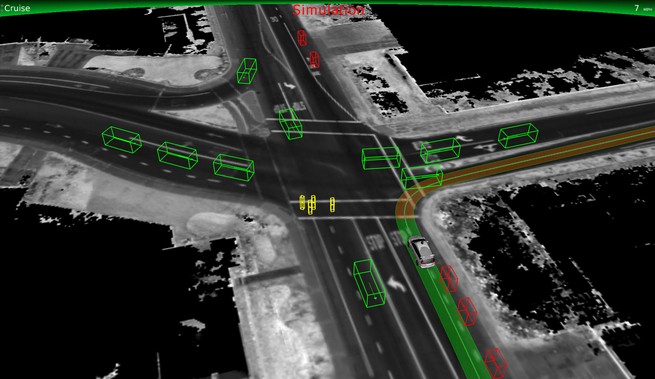
Not surprisingly, the hardest thing to simulate is the behavior of the other people. It’s like the old parental saw: “I’m not worried about you driving. I’m worried about the other people on the road.”
“Our cars see the world. They understand the world. And then for anything that is a dynamic actor in the environment—a car, a pedestrian, a cyclist, a motorcycle—our cars understand intent. It’s not enough to just track a thing through a space. You have to understand what it is doing,” Dmitri Dolgov, Waymo’s vice president of engineering, tells me. “This is a key problem in building a capable and safe self-driving car. And that sort of modeling, that sort of understanding of the behaviors of other participants in the world, is very similar to this task of modeling them in simulation.”
There is one key difference: In the real world, they have to take in fresh, real-time data about the environment and convert it into an understanding of the scene, which they then navigate. But now, after years of work on the program, they feel confident that they can do that because they’ve run “a bunch of tests that show that we can recognize a wide variety of pedestrians,” Stout says.
So, for most simulations, they skip that object-recognition step. Instead of feeding the car raw data it has to identify as a pedestrian, they simply tell the car: A pedestrian is here.
At the four-way stop, Kolarov is making things harder for the self-driving car. She hits V, a hot key for vehicle, and a new object appears in Carcraft. Then she mouses over to a drop-down menu on the righthand side, which has a bunch of different vehicle types, including my favorite: bird_squirrel.
The different objects can be told to follow the logic Waymo has modeled for them or the Carcraft scenario builder can program them to move in a precise way, in order to test specific behaviors. “There’s a nice spectrum between having control of a scenario and just dropping stuff in and letting them go,” Stout says.
Once they have the basic structure of a scenario, they can test all the important variations it contains. So, imagine, for a four-way stop, you might want to test the arrival times of the various cars and pedestrians and bicyclists, how long they stop for, how fast they are moving, and whatever else. They simply put in reasonable ranges for those values and then the software creates and runs all the combinations of those scenarios.
They call it “fuzzing,” and in this case, there are 800 scenarios generated by this four-way stop. It creates a beautiful, lacy chart—and engineers can go in and see how different combinations of variables change the path that the car would decide to take.
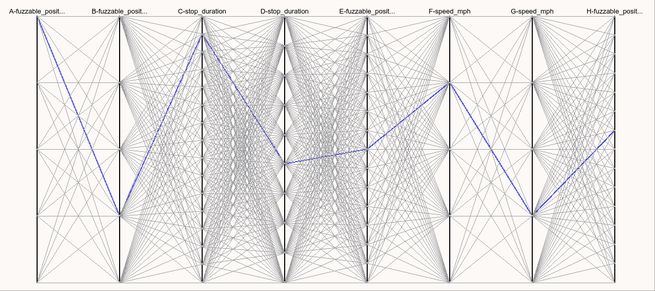
The problem really becomes analyzing all these scenarios and simulations to find the interesting data that can guide engineers to be able to drive better. The first step might just be: Does the car get stuck? If it does, that’s an interesting scenario to work on.
Here we see a video that shows exactly such a situation. It’s a complex four-way stop that occurred in real life in Mountain View. As the car went to make a left, a bicycle approached, causing the car to stop in the road. Engineers took that class of problem and reworked the software to yield correctly. What the video shows is the real situation and then the simulation running atop it. As the two situations diverge, you’ll see the simulated car keep driving and then a dashed box appear with the label “shadow_vehicle_pose.” That dashed box shows what happened in real life. To Waymo people, this is the clearest visualization of progress.
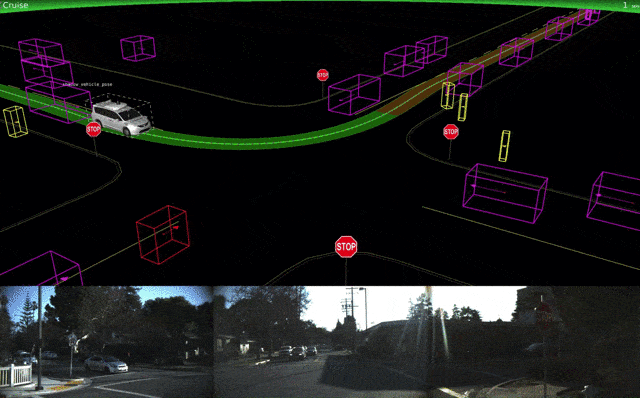
But they don’t just have to look for when the car gets stuck. They might want to look for too-long decision times or braking profiles outside the right range. Anything that engineers are working on learning or tuning, they will simulate looking for problems.
Both Stout and the Waymo software lead Dolgov stressed that there were three core facets to simulation. One, they drive a lot more miles than would be possible with a physical fleet—and experience is good. Two, those miles focus on the interesting and still-difficult interactions for the cars rather than boring miles. And three, the development cycles for the software can be much, much faster.
“That iteration cycle is tremendously important to us and all the work we’ve done on simulation allows us to shrink it dramatically,” Dolgov told me. “The cycle that would take us weeks in the early days of the program now is on the order of minutes.”
Well, I asked him, what about oil slicks on the road? Or blown tires, weird birds, sinkhole-sized potholes, general craziness. Did they simulate those? Dolgov was sanguine. He said, sure, they could, but “how high do you push the fidelity of the simulator along that axis? Maybe some of those problems you get better value or you get confirmation of your simulator by running a bunch of tests in the physical world.” (See: Castle.)
The power of the virtual worlds of Carcraft is not that they are a beautiful, perfect, photorealistic renderings of the real world. The power is that they mirror the real world in the ways that are significant to the self-driving car and allow it to get billions more miles than physical testing would allow. For the driving software running the simulation, it is not like making decisions out there in the real world. It is the same as making decisions out there in the real world.
And it’s working. The California DMV requires that companies report the miles that they’ve driven autonomously each year along with disengagements that test drivers make. Not only has Waymo driven three orders of magnitude more miles than anyone else, but their number of disengagements have fallen quickly.
Waymo drove 635,868 autonomous miles from December 2015 to November 2016. In all those miles, they only disengaged 124 times, for an average of about once every 5,000 miles, or 0.20 disengagements per 1,000 miles. The previous year, they drove 424,331 autonomous miles and had 272 disengagements, for an average of once every 890 miles, or 0.80 disengagements per 1,000 miles.
While everyone takes pains to note that these are not exactly apples-to-apples numbers, let’s be real here: These are the best comparisons we’ve got and in California, at least, everybody else drove about 20,000 miles. Combined.
The tack that Waymo has taken is not surprising to outside experts. “Right now, you can almost measure the sophistication of an autonomy team—a drone team, a car team—by how seriously they take simulation,” said Chris Dixon, a venture capitalist at Andreessen Horowitz who led the firm’s investment in the simulation company Improbable. “And Waymo is at the very top, the most sophisticated.”
I asked Allstate Insurance’s head of innovation, Sunil Chintakindi, about Waymo’s program. “Without a robust simulation infrastructure, there is no way you can build [higher levels of autonomy into vehicles].” he said. “And I would not engage in conversation with anyone who thinks otherwise.”
Other self-driving car researchers are also pursuing similar paths. Huei Peng is the director of Mcity, the University of Michigan’s autonomous- and connected- vehicle lab. Peng said that any system that works for self driving cars will be “a combination of more than 99 percent simulation plus some carefully designed structured testing plus some on-road testing.”
He and a graduate student proposed a system for interweaving road miles with simulation to rapidly accelerate testing. It’s not unlike what Waymo has executed. “So what we are arguing is just cut off the boring part of driving and focus on the interesting part,” Peng said. “And that can let you accelerate hundreds of times: A thousand miles becomes a million miles.”
What is surprising is the scale, organization, and intensity of Waymo’s project. I described the structured testing that Google had done to Peng, including the 20,000 scenarios that had made it into simulation from the structured testing team at Castle. But he misheard me and began to say, “Those 2,000 scenarios are impressive,”—when I cut in and corrected him—“It was 20,000 scenarios.” He paused. “20,000,” he said, thinking it over. “That’s impressive.”
And in reality, those 20,000 scenarios only represent a fraction of the total scenarios that Waymo has tested. They’re just what’s been created from structured tests. They have even more scenarios than that derived from public driving and imagination.
“They are doing really well,” Peng said. “They are far ahead of everyone else in terms of Level Four,” using the jargon shorthand for full autonomy in a car.
But Peng also presented the position of the traditional automakers. He said that they are trying to do something fundamentally different. Instead of aiming for the full autonomy moon shot, they are trying to add driver-assistance technologies, “make a little money,” and then step forward toward full autonomy. It’s not fair to compare Waymo, which has the resources and corporate freedom to put a $70,000 laser range finder on top of a car, with an automaker like Chevy that might see $40,000 as its price ceiling for mass-market adoption.
“GM, Ford, Toyota, and others are saying ‘Let me reduce the number of crashes and fatalities and increase safety for the mass market.’ Their target is totally different,” Peng said. “We need to think about the millions of vehicles, not just a few thousand.”
And even just within the race for full autonomy, Waymo now has more challengers than it used to, Tesla in particular. Chris Gerdes is the director of the Center for Automotive Research at Stanford. Eighteen months ago, he told my colleague Adrienne LaFrance that Waymo “has much greater insight into the depth of the problems and how close we are [to solving them] than anyone else.” When I asked him last week if he still thought that was true, he said that “a lot has changed.”
“Auto manufacturers such as Ford and GM have deployed their own vehicles and built on-road data sets,” he said. “Tesla has now amassed an extraordinary amount of data from Autopilot deployment, learning how the system operates in exactly the conditions its customers experience. Their ability to test algorithms on board in a silent mode and their rapidly expanding base of vehicles combine to form an amazing testbed.”
In the realm of simulation, Gerdes said that he had seen multiple competitors with substantial programs. “I am sure there is quite a range of simulation capabilities but I have seen a number of things that look solid,” he said. “Waymo no longer looks so unique in this respect. They certainly jumped out to an early lead but there are now a lot of groups looking at similar approaches. So it is now more of a question of who can do this best.”
This is not a low-stakes demonstration of a neural network’s “brain-like” capacities. This is making a massive leap forward in artificial intelligence, even for a company inside Alphabet, which has been aggressive in adopting AI. This is not Google Photos, where a mistake doesn’t mean much. This is a system that will live and interact in the human world completely autonomously. It will understand our rules, communicate its desires, be legible to our eyes and minds.
Waymo seems like it has driving as a technical skill—the speed and direction parts of it—down. It is driving as a human social activity that they’re working on now. What is it to drive “normally,” not just “legally”? And how does one teach an artificial intelligence what that means?
It turns out that building this kind of artificial intelligence does not simply require endless data and engineering prowess. Those are necessary, but not sufficient. Instead, building this AI requires humans to sync with the cars, understanding the world as they do. As much as anyone can, the drivers out at Castle know what it is to be one of these cars, to see and make decisions like them. Maybe that goes both ways, too: The deeper humans understand the cars, the deeper the cars understand humans.
A memory of a roundabout in Austin becomes a piece of Castle becomes a self-driving car data log becomes a Carcraft scenario becomes a web of simulations becomes new software that finally heads back out on a physical self-driving car to that roundabout in Texas.
Even within the polygon abstraction of the simulation the AI uses to know the world, there are traces of human dreams, fragments of recollections, feelings of drivers. And these components are not mistakes or a human stain to be scrubbed off, but necessary pieces of the system that could revolutionize transportation, cities, and damn near everything else.